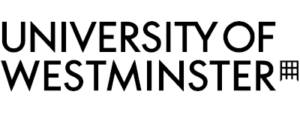
Summary: Within the older British Sign Language community, dementia can show itself as changes in the way someone signs – but these subtle changes are hard to spot by those who don’t use sign language. Dr Anastasia Angelopoulou and her team have developed an automated machine learning tool that can spot these changes. The tool will help identify the early stages of dementia among older users of sign language – ensuring they get the right support quicker.
We wanted to know whether it’s possible to detect the early stages of dementia among older BSL users in an automated way – without the need for sign language translation or interpretation.
Dr Anastassia Angelopoulou, Principal Investigator
Changes in memory or cognitive decline indicating the early stages of dementia are often difficult to identify in people who are deaf, especially if their family and carers have limited or no British Sign Language (BSL) experience. When carers do notice a deterioration in signing or communication, it’s often attributed to their deafness and age.
A very limited number of healthcare professionals are experienced in working with the sign language community and understand enough BSL to detect changes that could be a sign of dementia. As a result, older BSL users are less likely to get the help and extra support they need when going through the early stages of dementia.
Creating a screening tool for dementia in older deaf users of BSL
We wanted to know whether it’s possible to detect the early stages of dementia among older BSL users in an automated way, without the need for sign language translation or interpretation. This would allow healthcare professionals with no BSL experience to screen for the disease.
To understand how signing changes when someone has dementia, we analysed videos of BSL users from UCL’s Deafness, Cognition and Language (DCAL) research data archives. We also filmed interviews with 40 older signers – including healthy older people and those with mild cognitive impairment or dementia. A psychologist from UCL – who is a deaf, native signer – helped to carry out the interviews by going to deaf clubs, retirement communities and care homes. While we were filming, there was widespread interest in the project from the deaf community, with many asking us to come back to share our results.
BSL users with dementia have specific variations in how they sign and my team designed a framework to track these differences in real-time. Our tool tracks hand trajectory, facial expressions and elbow movement. Data about these three features are extracted in real time from videos and used to determine whether the individual might have dementia.
A successful proof-of-concept tool
The framework uncovered that BSL users with early stages of dementia sign slower, have more pauses, sign in a smaller area in front of their bodies and display more limited facial expressions.
Taking a machine learning approach, we taught the tool to distinguish between normal variation among older, healthy BSL users and atypical changes that could be a sign of early stages of dementia. As a result, our tool has an almost 90% accuracy in identifying BSL users who have features of signing associated with mild cognitive impairment or dementia.
There was widespread interest in the project from the deaf community with many asking us to come back to share our results. Following delays due to COVID-19, we’re looking forward to presenting our final findings – travelling back to the places we filmed to share what we’ve learned.
Dr Anastasia Angelopoulou
Even though we built the tool using BSL it can be used with any sign language because it doesn’t rely on translation or interpretation of the language itself. This means it could also prove useful in identifying movement impairments associated with other neurological conditions such as Parkinson’s or following a stroke.
What’s happening next
Funding from the Dunhill Medical Trust enabled work on a stimulating project alongside colleagues with expertise in a number of different fields. This award has led to strong working relationships and new friendships among the project team and partner institutions. Together we’re preparing new proposals to test the tool with more data and in real-life, clinical settings.
Since the tool uses machine learning, we’re also hoping to improve transparency. It’s important that the tool can explain how and why it reached a decision. This would help healthcare professionals have better conversations with BSL users and their families during their diagnosis journey.
Throughout the project, we shared our progress with the academic and BSL communities; for example, using a dedicated project website and attending events and conferences. We’ve also made the software code for the tool openly available for other interested researchers to use via GitHub.
Following delays caused by COVID-19, we’re looking forward to presenting our final findings – travelling back to the places we filmed to share what we’ve learned. We’re also preparing a BSL version of the project’s final report for the DCAL research centre website.
Find out more
You can read more about the project here including a short video of one of the research team talking about how the tool was developed.